NCWIT Selects 2025 AiC Collegiate Award Recipients
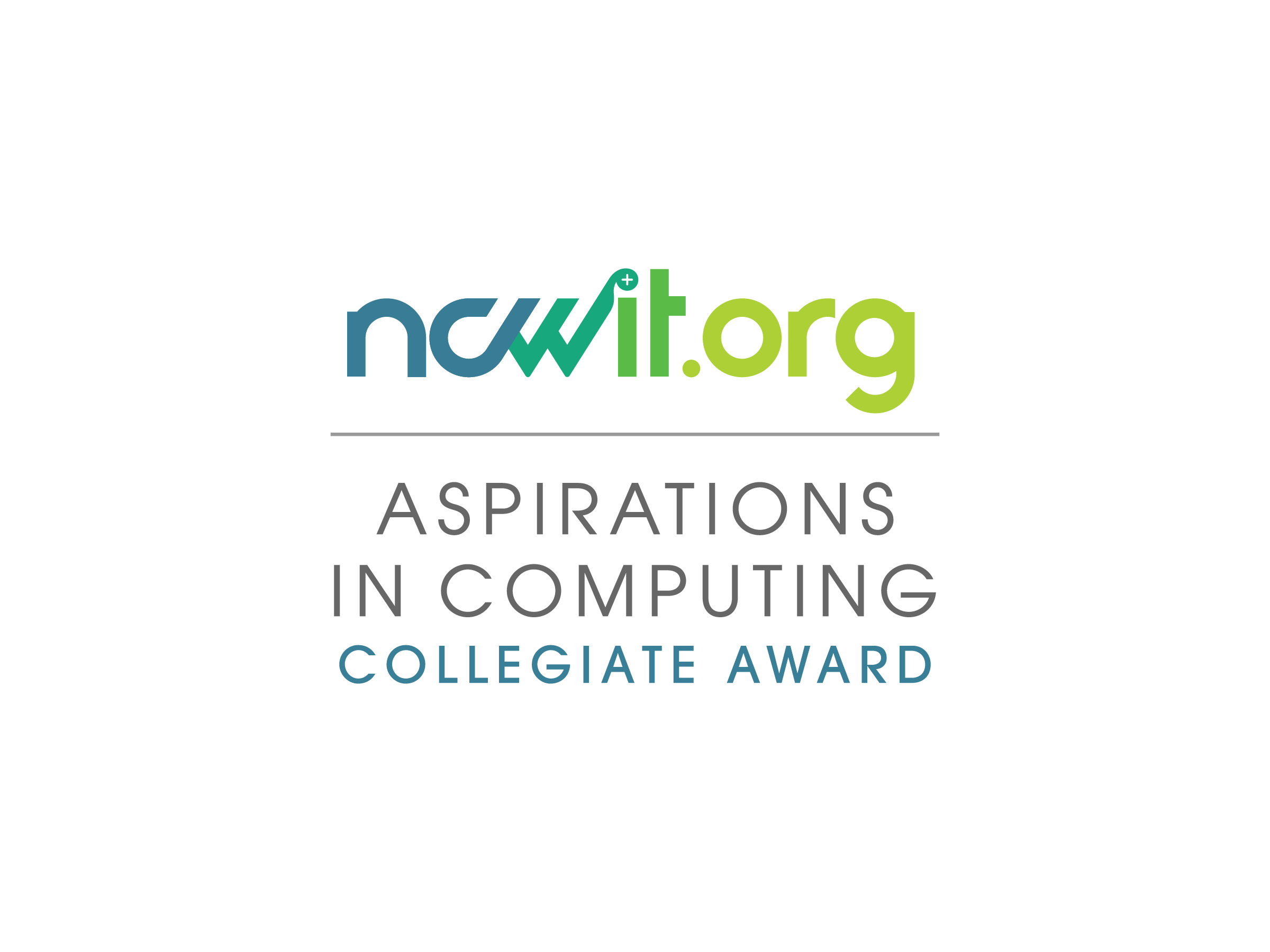
The NCWIT Aspirations in Computing (AiC) Program is pleased to announce the 2025 NCWIT AiC Collegiate Award recipients, celebrating 12 undergraduate and graduate students from 12 academic institutions nationwide. The award recognizes technical contributions to projects demonstrating a high innovation level and potential impact.
The multi-tiered award structure includes Winners and Honorable Mentions.
The entire NCWIT AiC program platform is supported generously by Apple. AiC also receives support for specific national program elements; the NCWIT AiC Collegiate Award is sponsored by Bank of America.
Winners
Mariam Ali | Class of 2025 at De Anza College
Achieving Equity in Maternal Health: A Novel Deep Learning and Generative AI Platform to Reduce Mortality and Improve Patient Care
This research project aims to develop a digital healthcare platform utilizing machine learning, cloud computing, and generative AI to enhance maternal health outcomes and reduce barriers in public healthcare. With a staggering number of women globally dying from preventable pregnancy-related issues, particularly in the U.S., where maternal mortality rates are highest among developed nations, there is an urgent need for innovative solutions.
Key features include Data-Driven Diagnostics, which utilizes deep learning to monitor vital health metrics and recognize early signs of conditions like preeclampsia and gestational diabetes. The application also bridges communication gaps, captures critical health data, and integrates medical records from various hospitals. Additionally, it offers solutions to navigate regulatory challenges while ensuring patient information remains secure and compliant. A pilot program conducted in collaboration with multiple hospitals revealed that healthcare professionals believe this tool will significantly improve patient care processes. With a deep learning model achieving a 93% accuracy in predicting maternal health complications, the project demonstrates promising potential for positive impact in maternal healthcare.
Tarushii Goel | Class of 2026 at Massachusetts Institute of Technology (MIT)
Every year, over 5.4 million people are diagnosed with basal and squamous cell cancer. Mohs Micrographic Surgery (MMS) is a precise treatment method that removes cancerous tissue layer by layer, involving close collaboration between surgeons and pathologists. However, the procedure’s effectiveness can be compromised by challenges like limited time for analysis, the complexity of tissue samples, the availability of expert pathologists, and difficulty in mapping findings to the resection site.
To address these issues, they developed ArcticAI, an artificial intelligence platform designed to enhance tissue preparation, histological inspection, and tumor mapping during MMS. ArcticAI streamlines the work of histotechnicians by using 3D modeling techniques and smart recommendations to expedite processing. It also employs sophisticated graph neural networks for efficient pathologic analysis and generates easy-to-understand pathology reports for surgeons in real-time or post-operatively.
By increasing the likelihood of complete tumor removal in a single surgery, ArcticAI minimizes invasiveness and decreases the need for follow-up procedures, potentially reducing cancer recurrence rates from over 15% to below 1%. This technology promises to broaden the feasibility of Mohs surgery for more complex tumors beyond skin cancers.
Vidya Srinivas | Class of 2028 at the University of Washington - Seattle
▶️ Knowledge Boosting During Low-Latency Inference
Understanding speech in noisy environments can be challenging, especially for individuals who are deaf, hard of hearing, neurodivergent, or have audio processing disorders. Conventional hearing aids amplify background noise, while headphones may suppress important voices. This project introduces a novel approach called knowledge boosting, which combines resource-constrained devices, like smart earbuds and glasses, with larger remote models through time-delayed collaboration for real-time audio enhancement. By utilizing source separation and target speech extraction techniques, they demonstrate how a smaller on-device audio deep learning model can effectively receive hints from a larger remote model, processing audio in real-time while minimizing latency and power consumption.
Their results show significant performance improvements, particularly when the gap between model sizes is wide, showcasing the potential for low-latency applications. This framework aims to enhance selective hearing and improve interactions in noisy environments without compromising user data privacy. By integrating this technology into everyday devices, they strive to make advanced audio processing accessible, helping users focus on desired sounds and experiences.
Honorable Mentions
Oghenemaro Anuyah | Class of 2025 at the University of Notre Dame
This project addresses the knowledge management challenges of community social service workers (CSWs) supporting vulnerable populations, such as those facing housing or food insecurity. Many CSWs rely on outdated systems that hinder efficient information sharing, impacting their ability to respond to urgent needs. This project explores how technology, specifically large language models (LLMs), can enhance their work while considering ethical implications.
Using a community-based participatory design approach, they organized workshops where CSWs collaborated as co-designers, sharing their experiences and aspirations. To accommodate participants with limited exposure to advanced AI tools, they employed accessible methods like speculative design activities to facilitate understanding and encourage participation. This co-design process resulted in an LLM-powered tool tailored to CSWs' needs, integrating with existing knowledge management platforms to improve information organization and sharing across networks.
By prioritizing the voices of those impacted, the project aims to create effective and meaningful tools, addressing critical gaps in knowledge management and fostering better outcomes for both CSWs and the communities they serve.
Etasha Donthi | Class of 2027 at the University of California - Berkeley
Livity
Livity is a pioneering tech startup founded to combat the mental health crisis and prevent suicide through artificial intelligence. By leveraging cutting-edge technology, Livity seeks to amplify the voices of those in need, connecting them with critical mental health resources before it's too late. It aims to give voice to those feeling unheard, particularly individuals who express their struggles on anonymous platforms like Reddit and X, where their posts often go unnoticed.
Livity employs an advanced AI algorithm to detect suicidal ideation in social media posts by analyzing sentiment and emotional cues to detect suicidal ideation in social media posts by analyzing the sentiment and emotion conveyed through text. Using advanced machine learning techniques like tokenization, hyperparameter tuning, and natural language processing models such as BERT and Transformers, Livity's algorithm parses language as a dynamic, living medium that carries deep emotional meaning. It identifies subtle patterns in word choice, tone, and context to flag potential cries for help that would otherwise remain hidden.
More than just a technical achievement, Livity represents a commitment to ensuring that no cry for help goes unheard by fostering a compassionate and urgent societal approach to mental health.
Tanvi Ganapathy | Class of 2026 at California Institute of Technology
Biophysical Study on the Kinetics of Cooperative DNA Hybridization
This project explores the advancements in DNA-based neural networks for artificial intelligence tasks, focusing on the winner-take-all (WTA) mechanism, which relies on cooperative DNA hybridization. Previous models faced challenges with unfair competition during the annihilation of input signals due to issues like reversible reactions and synthesis errors. Their biophysical study examines the kinetics of cooperative DNA hybridization, aiming to enhance the annihilation mechanism for improved classification accuracy.
They discovered that adjusting the molecular mechanics can reduce reversibility and identified how specific DNA sequence changes influence fair competition in the WTA process. By minimizing synthesis errors, they can further correct biases between inputs. Utilizing computational simulations, they calculated energy differences based on DNA sequence variations and developed a sequence-specific model for annihilation kinetics.
This model allows for the design of signal strands that foster fair competition in WTA, leading to improved performance in DNA neural networks. Ultimately, their findings provide insights into annihilation kinetics and pave the way for optimizing molecular design, enhancing the overall robustness of molecular pattern recognition.
Remley Hooker | Class of 2028 at Purdue University
EyeCanCode addresses the barriers that prevent disabled individuals from entering the tech industry, where they represent only 11% of the workforce despite making up nearly one-fifth of the U.S. population. Many existing tech education platforms are not designed for children with disabilities, especially those with mobility challenges. EyeCanCode eliminates traditional input methods by using voice recognition and gaze-based interaction, allowing kids to engage with coding without the need for keyboards or mice. This innovative approach enables children with physical disabilities to explore technology independently.
The platform fosters a belief in their potential to become future coders and innovators, combating feelings of isolation and self-doubt often faced by these children. Designed with Universal Design for Learning principles, EyeCanCode offers lessons in various formats and breaks tasks into manageable steps, accommodating diverse learning styles. By integrating assistive technologies into a fun learning environment, EyeCanCode empowers children with disabilities to pursue tech careers and achieve their goals, demonstrating that they can accomplish anything they set their minds to, regardless of their challenges.
Zulekha Karachiwalla | Class of 2027 at Carnegie Mellon University
▶️ Towards Robot-Assisted Wound Care
Chronic wounds affect an estimated 8.4 million Americans and incur annual healthcare costs of $22 billion. As the population ages, addressing this growing issue is critical, especially amidst a nursing shortage that impacts care quality and leads to nurse burnout. To tackle these challenges, they launched a two-phase project focusing on robotic solutions in wound care.
Initially, they conducted a mixed-methods study to identify opportunities for robotic assistance, emphasizing a user-centered approach through ethnographic research and collaboration with nursing professionals, HCI researchers, and engineers. This led to a focused observational study, producing innovative insights such as a task-based wound care taxonomy and evidence-based design recommendations.
In the second phase, they developed a specialized robotic system with a novel end-effector for wound dressing. This solution directly stems from their initial findings, aiming to alleviate nurses' workload while ensuring quality patient care.
Stephanie Nawas | Class of 2026 at the University of California - Davis
▶️ Provable Repair of Vision Transformers
What do we do when self-driving cars are unable to identify traffic signals in extreme weather conditions? How can we improve medical imaging that frequently misidentifies cancerous cells? These questions continue to arise as the reliance on image recognition in our day-to-day life further expands. One solution is to use Vision Transformers, a leading tool for image recognition. But Vision Transformers can still make mistakes, which can be problematic in safety-critical situations. This project, titled Provable Repair of Vision Transformers (PRoViT), proposes a method that ensures Vision Transformers correctly classify images within a specific set. PRoViT works by making minimal adjustments to the model's parameters, so it doesn't negatively affect images that were already correctly classified. PRoViT has the ability to provably correct thousands of images at a time, so our approach is scalable to real-world problems. PRoViT is a generalized approach, meaning that it can improve the safety of Vision Transformers in fields such as autonomous vehicles and medical diagnosis. They published PRoViT in the International Symposium on AI Verification (SAIV) conference in 2024 (https://link.springer.com/chapter/10.1007/978-3-031-65112-0_8).
Lucy Rubin | Class of 2025 at Macalester College
Blocks4All is an accessible iPad app designed to teach children the fundamentals of computer programming, particularly benefiting those who are blind or have low vision. Traditional coding often presents visual barriers, but Blocks4All uses Apple’s accessibility features, including screen readers, switch control, and voice activation, allowing users to learn coding without relying solely on visual cues. The app accommodates children with various abilities, employing high contrast images and simplified gestures for ease of use.
Unlike typical coding apps that display results visually, Blocks4All can be paired with Wonder Workshop’s Dash Robot. This programmable robot provides tactile, auditory, and visual feedback, allowing users to engage through touch and sound. Blind or low-vision children can feel the robot as it moves, while those with motor impairments can see its effects in action. This interactive experience not only promotes learning but also fosters an inclusive environment where children can explore coding and technology together.
Richael Saka | Class of 2027 at Harvard University
Sana is a public health tool aimed at reducing breast cancer disparities in underserved rural areas of Mexico. It combines environmental risk awareness, personalized risk assessments, and culturally sensitive education to help both residents and policymakers make informed health decisions. The mobile app provides real-time data on environmental risks, such as air and water quality, while assessing individual breast cancer risk through user surveys. For policymakers, a web dashboard visualizes regional risk hotspots by integrating demographic insights with environmental data.
Powered by a machine learning algorithm, Sana predicts breast cancer risks using various factors, including air quality and lifestyle. The project also tackles challenges like the lack of centralized health data and cultural stigmas surrounding breast cancer, ensuring educational content resonates with diverse communities. To enhance accessibility, offline components like paper surveys and pamphlets are utilized. Through partnerships with local NGOs and community outreach, Sana not only raises awareness about the environmental contributors to breast cancer but also informs policies and programs, fostering a commitment to health and empowerment throughout Mexico.
Shreya Sreekantham | Class of 2025 at Northeastern University
Detecting and Eliminating Cardiac Artifact from Endovascular EEG Signals
Paralysis affects over 5.4 million people in the U.S., often leaving patients completely unable to communicate. The Stentrode, a novel brain-computer interface, offers a minimally invasive solution by recording brain signals through an implant in a vein. The first clinical trials of this device aim to distinguish between brain signals when the patient is resting versus attempting to move their ankles. Machine learning classifiers can then translate these signals into computer inputs. However, the placement of the implant and transmitter causes cardiac noise to dominate the signal, making it challenging to analyze the signal and limiting the accuracy of machine learning classifiers to 70%.
To address this, they developed an automated method using independent component analysis to detect and remove cardiac noise from the brain signals. They also reconstructed and analyzed the cardiac activity separately, identifying features like increased heart rate during movement attempts compared to rest periods. They discovered features such as increased heart rate during movement attempts compared to rest periods. Incorporating these features into the classifier improved its accuracy to more than 90%. This advancement brings us closer to enabling effective communication for paralyzed patients, significantly enhancing their quality of life.